Scaling GPS Crowdsourced Data for Visitation Counts
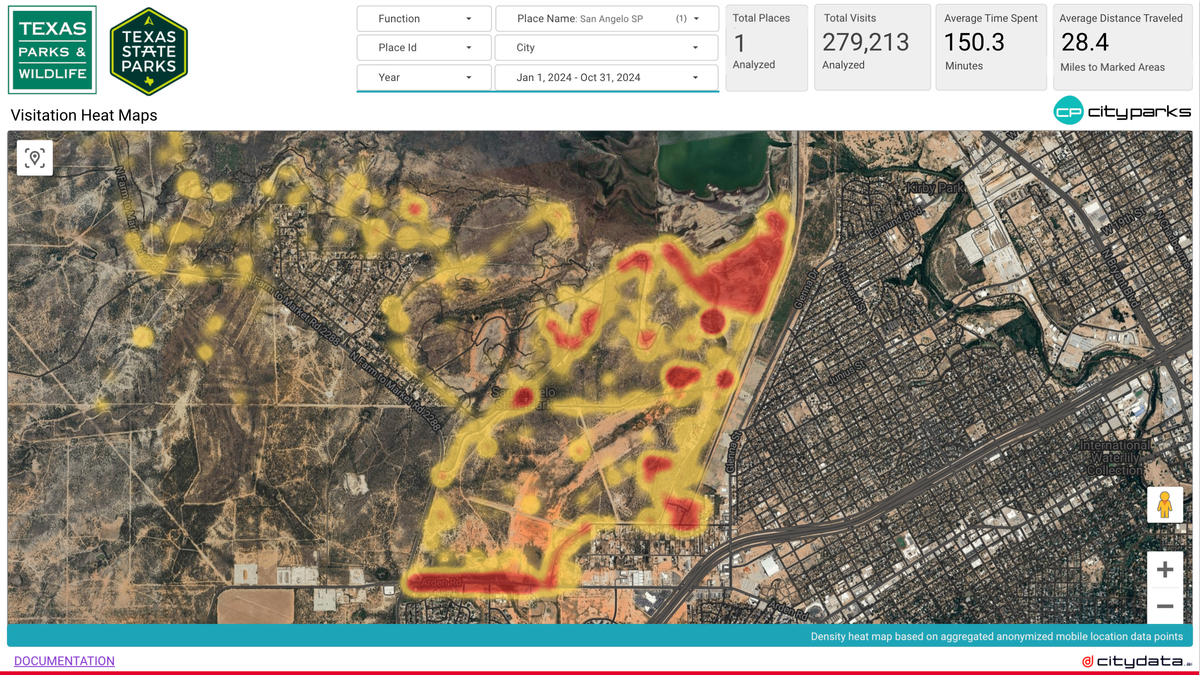
Understanding visitation patterns to various points of interest (POIs) like parks, trails, downtowns, business districts, shopping malls, restaurants, and individual businesses is crucial for urban planning, economic development, and tourism. Anonymized GPS crowdsourced data offers a rich source of information for measuring these visits and dwell times. However, this data typically represents a sample of the population, not the entire population. Therefore, we need robust scale-up methodologies to extrapolate from the sample to represent the true visitation counts.
Why Scale-Up is Essential:
GPS data, while valuable, is often collected from a subset of individuals. This sample might not be representative of the overall population due to factors like smartphone ownership, app usage, and user demographics. Without scaling, we risk underestimating or misrepresenting actual visitation. Accurate visitation counts are essential for:
- Urban Planning: Understanding park usage, trail traffic, and downtown activity helps cities allocate resources effectively and plan for future development.
- Economic Development: Businesses can use visitation data to assess market potential, optimize operations, and make informed investment decisions.
- Tourism Development: Downtown zones and tourism agencies can target their outreach efforts more effectively by understanding tourist traffic patterns.
- Performance Measurement: Organizations can track the impact of events, promotions, or infrastructure improvements on visitation.
Common Scale-Up Approaches:
Several methods exist for scaling GPS crowdsourced data. Here are some common approaches:
- Ratio-Based Scaling: This method involves calculating a ratio between the number of visitors observed in the GPS data and a known population or benchmark for the area of interest. For example, if we know the total population of a census tract and we observe a certain number of visitors in our GPS data, we can calculate a scaling ratio and apply it to other observed data points. This is similar to the residential inference and daily ratio methods described in the previous blog post.
- Demographic Weighting: If the GPS data sample is biased towards certain demographics, we can apply weights to adjust for these biases. For example, if our sample overrepresents younger individuals, we can weight the data to better reflect the age distribution of the overall population.
- Trip Chaining and Expansion: This approach involves analyzing trip patterns to infer visits to multiple locations. If a device is observed at a park and then later at a restaurant, we can infer a visit to both locations, even if the GPS data is only available for a portion of the trip. Expansion factors are then used to account for trips not captured in the data.
- Hybrid Approaches: Often, a combination of these methods is used to achieve the most accurate results. For instance, we might use ratio-based scaling combined with demographic weighting to adjust for both sample size and demographic biases.
Confidence Scores for Scale-Up:
Measuring the confidence in our scaled estimates is crucial. Several factors influence confidence:
- Sample Size: Larger sample sizes generally lead to higher confidence.
- Representativeness: A sample that accurately reflects the overall population will yield more reliable results.
- Data Quality: Accurate and reliable GPS data is essential.
- Methodology: The chosen scale-up methodology can impact confidence.
Confidence scores can be calculated using statistical methods, such as calculating confidence intervals based on the variance in the data and the sample size. We can also use techniques like bootstrapping to estimate the uncertainty in our scaled estimates.
Drawbacks of Scale-Up:
Scaling GPS data has limitations:
- Bias: Even with weighting, it's challenging to completely eliminate all biases.
- Data Sparsity: In some areas, GPS data might be sparse, making it difficult to generate reliable estimates.
- Privacy Concerns: Aggregating and anonymizing GPS data is essential to protect individual privacy.
- Accuracy of Ground Truth Data: When calibrating with ground truth data, the accuracy of that data itself is a factor.
Generative AI and Scale-Up:
Generative AI could potentially play a role in improving scale-up methodologies. For example:
- Synthetic Data Generation: Generative AI could be used to create synthetic GPS data that better represents the overall population, addressing issues of sample bias.
- Improved Imputation: AI models could be trained to impute missing data points more accurately, improving the completeness of the dataset.
- Pattern Recognition: Generative AI could be used to identify complex patterns in visitation behavior that are difficult to detect with traditional methods.
However, using generative AI for scale-up requires careful consideration of potential biases in the training data and ensuring that the generated data is used responsibly and ethically. It's crucial to validate the results of any AI-driven scale-up method against ground truth data and other independent sources.
By carefully considering the various scale-up approaches, understanding their limitations, and exploring new possibilities with AI, we can leverage the power of anonymized GPS crowdsourced data to gain valuable insights into visitation patterns and make better data-driven decisions.
About CITYDATA.ai
CITYDATA.ai brings mobility big data + AI to make cities smarter, sustainable, and more resilient. We provide insights about people counts, density patterns, movement trends, economic impact, and community engagement.
Founded in 2020 in San Francisco, California, CITYDATA.ai provides fresh, accurate, daily insights that are essential for smart city programs, economic development, urban planning, mobility and transportation, tourism, parks and recreation, disaster mitigation, sustainability, and resilience.
You can reach us via email at business@citydata.ai if you’d like to discuss your data needs and use cases. You can also follow the company on Linkedin, and the UniverCity.ai blog to stay updated on the newest innovations in big data and AI for the public sector.